Symbiosis between humans and AI6 min read
Reading Time: 5 minutesReading Time: 5 minutesWhat are the fundamental differences in the learning capabilities of machines and humans? The learning process in machines (AI), primarily driven by algorithms, excels in handling vast amounts of data with incredible speed and efficiency. Machine learning thrives on pattern recognition and consistency, operating within the scope of its programming to analyze and make predictions based on large datasets. This method, however, cannot sometimes grasp contextual nuances and make ethical judgments. In contrast, human learning is a multifaceted process involving cognitive understanding, emotional intelligence, and social interactions.
Humans can interpret complex contexts, make value-based decisions, and demonstrate creativity and adaptability in their learning. While machines provide precision and volume, human wisdom adds depth, emotional understanding, and creative problem-solving. These contrasting capabilities highlight the unique strengths of both machine and human learning, each playing pivotal roles in their respective domains.
The phenomenon of humans learning from machines they interact with represents a significant shift in the traditional knowledge acquisition and application dynamics. In this evolving landscape, the interaction with intelligent systems is not just a one-way process where humans input data and receive output. Instead, it’s an interactive, continuous learning experience. Advanced machine learning systems expose humans to new patterns, insights, and ways of problem-solving that were previously unrecognized as they engage with them. This exposure can lead to a profound expansion of human understanding and capabilities in various domains, ranging from data analysis to complex decision-making.
Human-machine symbiosis: the future of intelligence
Jörg’s theory describes reciprocal learning as a dynamic, autonomous process with significant long-term impacts. Professor David Schwartz, from Bar-Ilan University, focuses on the continuous learning between humans and machines. He co-authored two articles, including “Reciprocal Human Machine Learning (RHML)” with Dov Te’eni and Inbal Yahav, highlighting the importance of mutual learning in human-machine interactions as a macro-level approach. The second study actively proposes creating a reciprocal learning model and offers detailed insights into organizing the interaction between humans and machines.
The study details three key aspects (challenges) of reciprocal collaboration between AI and humans: Theory: Adapting reciprocal learning for human-machine use; Technology: Creating a prototype known as Fusion, designed to categorize text messages — an abstract configuration for a system that promotes joint continual learning of both human experts and ML models. Researchers apply it to inform the configuration’s development and demonstrate its performance. Design Principles: Guiding learning scenarios involving experts generating concepts and returning feedback. After each learning cycle, the human experts completed questionnaires about their learning experiences.
To tackle the challenges in the project, the team implemented two key strategies:
1. Conducted a functional analysis to identify the specific learning tasks to perform.
2. Made a communication analysis to determine the requirements for HMRL interaction.
Since 2018, they have conducted various case studies to explore further and enhance methods and systems of the analysis. The nine-member research team included qualitative research methods experts developing machine learning algorithms. They are experts in understanding how people think. Some were good at making computer programs, and others worked on designing how people and machines work together. Additionally, they collaborated with cybersecurity experts from a company specializing in Darknet communication analysis, providing real-world insights and applications.
This setup below demonstrates distinctive functions in the Learning Cycle, including human sense-making and machine classification with lexicon models enhanced by semantic similarities (Figure 1). It is a system designed to classify textual messages. One of the critical components of RHML is the use of lexicons and concept maps. Unlike interactions between humans with similar cognitive abilities, RHML (Reciprocal Human-Machine Learning) involves unique roles for humans and machines, aligning with their different capabilities.
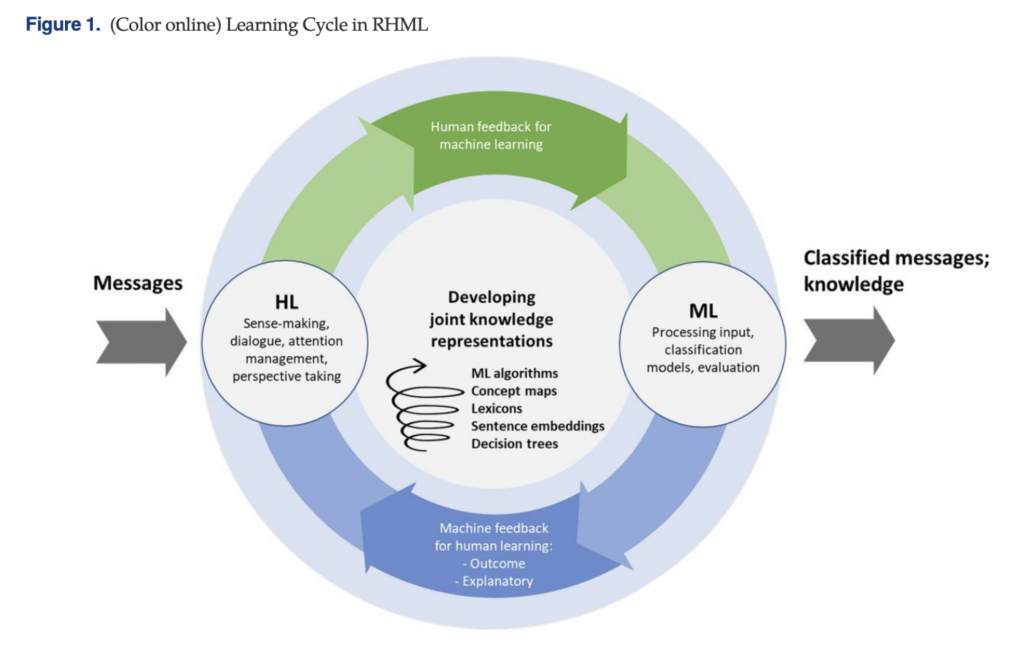
The ultimate goal of RHML is mutual improvement, with machines becoming more precise and humans enhancing their skills. Ethical considerations are central, as this reciprocal learning requires a deeper understanding of AI’s impact on humans and vice versa.
Are humans still essential in an AI-driven world?
RHML harnesses machine intelligence, focusing primarily on specific tasks and excelling in processing large volumes of data swiftly and efficiently. In contrast, traditional human learning offers a more holistic approach, seamlessly integrating cognitive and factual knowledge with emotional intelligence and experiential insight. While RHML relies on data-driven methodologies, human understanding adopts a more holistic approach, blending cognitive processes with experiences, emotions, and social interactions. Consequently, regarding information processing, machines in RHML are adept at handling vast quantities of data at high speeds. Still, human learning emphasizes depth, bringing a richer understanding and contextual interpretation to the information processed.
The central challenge in RHML lies in balancing contributions from both humans and machines and fostering a joint understanding. This paradigm aims to create a robust human-in-the-learning-loop (HITLL) framework, where humans and machines continuously learn and evolve through interaction.The concept emphasizes a two-way learning process between humans and machines.
In traditional machine learning, the learning process is primarily one-directional: machines learn from data provided by humans, and algorithms are improved based on human feedback and intervention. However, in RHML, the learning is reciprocal – not only do machines learn from humans, but humans also learn from machines. It’s a more symbiotic relationship between humans and AI, where each learns from and complements the other, leading to more effective and responsible use of AI technology.
Discover the advantages of integrating technology in the classroom by reading our previous note, “Why I am never teaching without an Artificial Intelligence Teaching Assistant again,” authored by Alex and Ilya.
Conclusion
As a result, this synergy between human and machine learning suggests a future where learning is a collaborative, interactive process, each continuously benefiting from and contributing to the other’s growth. As humans evolve in their understanding and use of technology, they can impart more sophisticated guidance to machines, offering more advanced insights and capabilities back to their human counterparts. The text concludes that integrating human-machine learning requires several steps. First, it involves understanding and managing the distinct roles of humans and machines. Next, it addresses the complexities of their communication. Finally, it focuses on developing knowledge representations compatible with both.
Machine learning models for text classification and human processes of understanding differ in how they use context to interpret texts, how they focus attention on information, and their capacity to explore and adopt different viewpoints. However, in Reciprocal Human-Machine Learning (RHML), interlinked learning approaches allow each to influence the other through cycles, contributing to the collective advancement of classification knowledge. The challenge involves balancing human and machine contributions to create a robust framework for continuous learning and evolution.
***
Reciprocal Human Machine Learning (RHML) bridges the gap between human and artificial intelligence, creating a symbiotic relationship where both entities learn from each other. The concept of Reciprocal Human Machine Learning (RHML) pioneered by Prof. David Schwartz and his colleagues represents a transformative approach in the realm of artificial intelligence and human-computer interaction. RHML, as a collaborative approach, signifies a paradigm shift where humans and machines are integral participants in a continuous learning process. The essence of RHML lies in its bidirectional nature of learning – a symbiotic relationship where machines learn from human feedback, and humans gain insights from machine predictions and analyses. This approach involves the creation of interactive systems that enable real-time feedback loops, fostering an environment where machine algorithms adapt dynamically to human interactions.