AI as a team player: exploring Collective Intelligence with Anita Williams Woolley5 min read
Reading Time: 4 minutesReading Time: 4 minutesIn this exploration, we discuss two insightful articles by Anita Williams Woolley, a distinguished figure in organizational behavior. The first, Articulating the role of Artificial Intelligence in Collective Intelligence: A Transactive Systems Framework, examines the intricate interplay between AI and collective intelligence (CI). The second article, Teaching agents to understand teamwork: Evaluating and predicting Collective Intelligence as a latent variable via Hidden Markov Models, takes a more analytical approach, using advanced modeling techniques to predict and enhance CI in team settings.
Collective Intelligence (CI) refers to the enhanced problem-solving and decision-making ability that emerges from groups’ collaboration and collaborative efforts, often supported by technology. Mentioned studies underscore the need for an adaptive system that fosters CI. Such a system moves beyond mere self-regulation, actively identifying roles where Artificial Intelligence (AI) can play a significant part. This reflects the shift in management theory, which now views organizations less as mechanistic entities and more as complex adaptive systems (Mathieu et al., 2019). This perspective is crucial in understanding how groups and organizations navigate and adapt in dynamic environments.
AI as the new team whisperer
The broader definition of intelligence, whether it pertains to individuals, collectives, or technological systems, encompasses the capability to achieve goals across various environments. In this context, memory, attention, and reasoning are pivotal for any intelligent system. These functions, crucial in human brain systems, are equally essential in other intelligent systems, including biological, technological (AI), and human-AI collaborations.
AI, particularly in its role in facilitating teamwork, is emerging as a transformative force. It extends beyond executing taskwork to aiding in navigating dynamic environments that lack traditional, well-defined organizational structures. These structures have historically been relied upon to augment humans’ bounded rationality. The development of artificial social intelligence (ASI) marks a significant leap in this direction. ASI’s ability to infer human mental states, encompassing goals, situational knowledge, beliefs, and emotions, can significantly enhance teamwork, especially in settings where human interactions are complex and multifaceted.
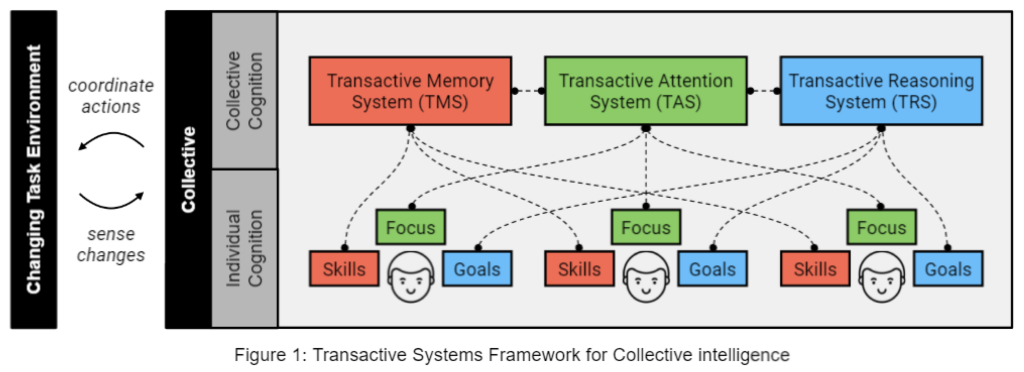
The “Transactive Systems Framework for Collective Intelligence” (Figure 1) focuses on how groups utilize and coordinate their cognitive resources and goals to enhance collective intelligence (CI). This framework comprises three systems: Transactive Memory System (TMS), Transactive Attention System (TAS), and Transactive Reasoning System (TRS).
TMS handles the understanding and management of each team member’s skills and knowledge, ensuring effective coordination and specialization.
TAS entails distributing and managing member attention, which is crucial for task accomplishment and efficient information processing.
TRS involves collective reasoning to align individual and group goals and motivations, that the team pursues valuable and achievable objectives.
Each system incorporates three processes: updating, allocation, and retrieval, which contribute to the development and efficient functioning of collective intelligence in various group settings.
Empathy by algorithm
In a world increasingly driven by technology, the collaboration between humans and artificial agents has become a focal point of research. Hidden Markov Models, where the states are not directly observable but can be inferred through visible outputs, are pivotal in studying collective intelligence (CI) within Human-Agent Teams (HATs). These models, renowned for their simplicity and effectiveness, are utilized extensively in fields like statistics, economics, and machine learning, mainly due to their ability to model systems with a “memoryless” property.
The core methodology of using Hidden Markov Models in this context involves interpreting various collaborative behaviors, such as the team’s collective effort, the utilization of individual skill sets, and the overall task strategy. By evaluating these factors, the model adeptly learns about the team’s CI, enabling it to predict future behavioral patterns and performance with remarkable accuracy. A study emphatically validated this predictive capability, involving human participants and a scripted agent in a search and rescue game scenario. The results of this study highlighted the model’s proficiency in accurately forecasting future states of CI in these dynamic team settings.
From adaptation to strategic enhancement
The implications of this research are significant, particularly in the realm of the AI’s integration in human collaboration. These findings underscore the potential of AI agents not only to adapt to human behavior but also to proactively contribute to enhancing team dynamics. The study suggests that the developed HMM approach allows for real-time monitoring and strategic interventions in team processes, potentially leading to increased CI and improved team performance.
However, the research also acknowledges certain limitations, such as simplifying the observation space and using pre-scripted agents, pointing towards areas for future improvement. Subsequent analysis is encouraged to focus on more nuanced intervention strategies and to consider additional variables like cognitive diversity and social intelligence of team members. Overall, the study represents a significant step in developing AI agents capable of enhancing human teamwork through strategic predictive modeling and interventions.
Conclusion
The insightful works of Anita Williams Woolley present a compelling intersection of artificial intelligence and collective intelligence, offering a transformative perspective on teamwork and organizational behavior. This integration promises to unlock new levels of efficiency, creativity, and effectiveness in collective endeavors, marking a pivotal moment in the evolution of CI. Through her “Transactive Systems Framework” and the use of Hidden Markov Models, Woolley demonstrates how AI can enhance human teamwork and decision-making.
The framework applies to diverse contexts, such as organizations, networks, and small groups, as it provides a structure for understanding and enhancing how groups coordinate cognitive resources and goals to develop collective intelligence. As shaped by these research advancements, the future of team collaboration and organizational effectiveness looks towards an era where AI and human intelligence coalesce seamlessly to achieve more significant collective goals.
***
Anita Williams Woolley, Associate Dean of Research and Professor at Carnegie Mellon University’s Tepper School of Business, is a well-known scholar in organizational behavior. With a Ph.D. from Harvard University, her research focuses on team collaboration and collective intelligence. Woolley has published extensively in top journals and received multiple academic honors. Her work is recognized globally, contributing significantly to understanding team dynamics and online collaboration.